Artificial Intelligence is undeniably reshaping pharmaceutical research and development. We hear daily about its power to accelerate drug discovery and personalize medicine. This is exciting. However, beyond these mainstream narratives lie complex, less-discussed challenges. These subtle, yet critical, hurdles span ethical considerations, regulatory frameworks, and practical implementation. Addressing them is key to truly unlocking AI’s vast potential. This post explores some trends and specific sub-topics that demand our attention, forming significant AI Healthcare Challenges.
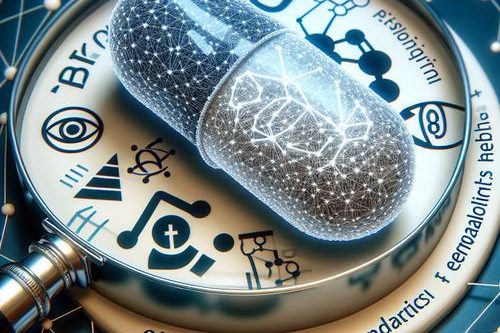
Nuances in AI Pharma Ethics
While discussions around AI ethics often focus on patient data privacy or job displacement, advanced pharma R&D presents its own unique ethical challenges. These are fundamental issues of AI Pharma Ethics that we must navigate carefully.
The Shadow of Bias in ‘Omics’ Data Lakes
‘Omics’ technologies – genomics, proteomics, metabolomics – generate vast datasets. AI models search through this data to identify novel drug targets or predict compound efficacy. But what if these foundational datasets are skewed? Historically, clinical research and genomic databases have overrepresented certain populations. This means AI trained on such data might inadvertently perpetuate health disparities. It could struggle to find targets for diseases prevalent in underrepresented groups. Or, it might optimize drug candidates primarily for the majority demographic. Companies like BenevolentAI leverage AI to analyze biomedical information for target identification. The success of such platforms inherently depends on the breadth and diversity of the data they access. Ensuring equitable data representation in AI training sets is a profound ethical imperative in early-stage R&D.
Generative AI’s “Explainability Gap” in Novel Molecule Design
Generative AI can design novel molecular structures from scratch. This capability promises to rapidly expand the chemical space for drug discovery. For instance, Insilico Medicine has demonstrated AI’s power in generating new drug candidates. However, a significant challenge is the ‘black box’ nature of some advanced AI. If an AI proposes a novel molecule, but we cannot fully understand *why* it chose that specific structure or predict its potential off-target effects based on the AI’s reasoning, how can we confidently proceed? This explainability gap has serious implications for AI Pharma Ethics. It impacts safety assessments, regulatory trust, and the ability to optimize these AI-generated leads. True innovation requires not just novelty, but also deep understanding.
Navigating Shifting Regulatory Sands for AI in R&D
Regulators worldwide are grappling with how to oversee AI in healthcare. Much focus is on patient-facing AI tools. However, AI used in the foundational R&D stages—long before human trials—presents distinct regulatory puzzles.
“Living Algorithms”: The Quest for Continuous Validation in Dynamic R&D
AI models in R&D are not static. They can learn and evolve as they process new data, improving their predictive accuracy over time. These are sometimes called “living algorithms.” This continuous learning is a huge advantage. But it’s a headache for traditional regulatory validation, which often relies on a fixed, point-in-time assessment. How can agencies like the FDA or EMA ensure the ongoing reliability and validity of an R&D AI tool that changes its parameters or even its core logic? While the FDA’s AI/ML-Based SaMD Action Plan provides a framework for adaptive algorithms, its primary focus is on Software as a Medical Device (SaMD) used in clinical care. Adapting such concepts for non-clinical R&D tools, which influence drug design years before patient exposure, is a key emerging challenge. This is one of the more nuanced AI Healthcare Challenges for regulatory bodies.
The Global Puzzle: Harmonizing AI Validation Standards for R&D
Pharmaceutical R&D is a global endeavor. A drug candidate might be discovered using AI tools in one country, tested preclinically with AI-driven insights in another, and eventually submitted for approval in many. However, there is currently no global consensus on how to validate AI tools used specifically in these early R&D phases. Different regions may have varying expectations—or no specific guidelines at all—for the evidence required to trust AI-derived R&D data. This lack of harmonization can create significant friction. It can slow down international collaborations and complicate regulatory submissions if the AI methodologies are not transparently documented and validated to a commonly accepted standard.
Clearing Real-World Hurdles: AI Implementation in the Lab
Beyond ethics and regulations, the practicalities of embedding AI into the intricate fabric of pharma R&D are often underestimated. These are the on-the-ground challenges that can make or break AI’s impact.
The Scarcity of “AI-Scientific Translators”
There’s a growing talent gap that isn’t just about hiring more data scientists or more biologists. The real bottleneck is finding and cultivating “AI-scientific translators.” These are individuals who possess deep expertise in both AI/machine learning and the specific scientific domain (e.g., pharmacology, medicinal chemistry). They can bridge the communication chasm between AI algorithms and wet-lab scientists. They translate complex AI outputs into testable hypotheses and actionable experimental designs. Without these translators, brilliant AI-generated insights might remain locked in computational models, failing to impact actual drug development pipelines. This human element is critical for effective AI implementation.
The “Last Mile”: AI Meets Real-World Lab Automation
AI can design an optimal experiment or suggest a synthesis pathway. But physically executing these plans in a laboratory involves another layer of complexity. Integrating AI decision-making engines with physical lab automation systems—robotics, liquid handlers, an_alytical instruments—is the “last mile” challenge. Companies like Opentrons offer accessible lab automation solutions. The next step is seamless AI control. Ensuring data integrity and quality as information flows from AI design, to robotic execution, and back to AI analysis is crucial. This involves robust data pipelines, standardized protocols, and systems that can handle the inevitable variability of real-world experiments. Overlooking this integration aspect can severely limit AI’s practical utility in accelerating R&D cycles.
Conclusion: Proactively Shaping AI’s Future in Pharma R&D
The journey of integrating AI into advanced pharma R&D is filled with immense promise, but also with unique, often subtle, challenges. From ensuring ethical data use in ‘omics’ research and demanding explainability in AI-driven design, to establishing agile regulatory frameworks for evolving algorithms and bridging the talent and lab integration gaps – these are not minor footnotes. They are central to the responsible and effective deployment of AI. These AI Healthcare Challenges require proactive, collaborative efforts from researchers, AI developers, ethicists, regulators, and pharmaceutical companies. By acknowledging and tackling these niche issues head-on, we can build a stronger foundation for AI Pharma Ethics and truly harness AI’s power to bring life-saving medicines to patients faster and more equitably. The future of medicine may well depend on how thoughtfully we navigate these unseen hurdles today.