The world of finance is changing fast. Gut feelings and basic spreadsheets are no longer enough for modern businesses. Companies need to see around corners and predict the future more clearly. How can they achieve this? The answer lies in artificial intelligence. Financial Planning and Analysis, or FP&A, is currently undergoing a major upgrade thanks to AI. AI is not just about automating old tasks. It’s unlocking entirely new ways to plan, predict, and make smarter financial decisions. This article dives deep into this transformation. We will explore exciting, emerging trends in AI in FP&A. We aim to look beyond the mainstream discussions. We’ll uncover how AI is truly shaping the next generation of finance, making it more predictive and insightful.
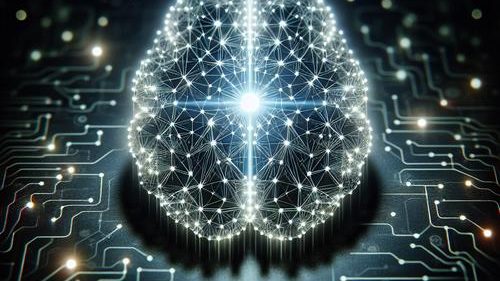
Trend 1: Personalised Financial Guidance and “Self-Driving” FP&A
FP&A teams traditionally spend a lot of time supporting other departments. They answer financial questions and build custom reports. This vital work can sometimes create bottlenecks. What if AI could assist with this, but in a much smarter, more proactive manner? Imagine AI acting as a personal finance co-pilot for every budget owner in your company. This vision is becoming a reality. It’s a key emerging trend in AI in FP&A: hyper-personalized financial guidance leading towards “self-driving” elements within finance functions.
This concept goes far beyond the basic automation of reports. We are talking about AI systems that understand context deeply. They learn individual departmental needs, historical performance, and strategic goals. These advanced AI tools could provide tailored advice directly to managers, in plain, understandable language. For example, a marketing manager might receive an alert on their dashboard: “Your Q3 social media campaign is currently 15% over budget on ad spend. Lead conversions are also 10% below target. Our AI financial forecasting model projects a negative ROI if current trends continue. Consider reallocating $5,000 from this campaign to the email marketing initiative. The email initiative is outperforming its targets by 20%.” This advice would be grounded in real-time data streams and sophisticated predictive analytics.
This personalisation shares complex financial knowledge. Department heads are experts in their own fields, but not always in finance. They receive clear, actionable insights directly from the AI. This setup empowers them to make faster, data-driven decisions at a local level. It also significantly frees up the central FP&A team. They can shift their focus from routine queries and manual report generation. Instead, they can concentrate on higher-value strategic initiatives. These include long-range strategic planning, merger and acquisition analysis, or developing new business models. Essentially, AI in FP&A becomes an embedded intelligence layer, enhancing decision-making across the entire organization.
While fully autonomous “self-driving” FP&A is still evolving, several companies are preparing the way. For instance, planning solutions from companies like Workday Adaptive Planning incorporate AI and machine learning. These help with sophisticated scenario modeling and anomaly detection within financial plans. Users can explore different business assumptions. The AI can then highlight potential issues or unseen opportunities. Such platforms are progressively moving towards providing more direct, personalized guidance. This reduces manual effort in tasks like variance analysis and forecasting updates. The journey is towards AI not just presenting data. It’s about AI interpreting data and suggesting concrete actions at a granular level.
Trend 2: AI in FP&A for Predictive Risk Modeling and Enhanced Resilience
Businesses today navigate a sea of constant uncertainty. Supply chains can fracture due to geopolitical events. New, agile competitors can disrupt established markets seemingly overnight. Economic policies shift, and climate change introduces unpredictable environmental impacts. Traditional FP&A methodologies attempt to model some of these risks. However, AI offers a vastly more powerful and dynamic toolkit to anticipate and manage them effectively. Using AI in FP&A for sophisticated predictive risk modeling and thereby enhancing organizational resilience is a vital emerging trend.
This capability goes far beyond running a few more “what-if” scenarios in a traditional spreadsheet model. It involves AI algorithms trained to look through and interpret huge, diverse datasets. Crucially, many of these datasets are unstructured. Think about global news articles, social media sentiment analysis, discussions on professional forums, new regulatory announcements, real-time shipping data, and even satellite imagery that might indicate resource availability or impending weather patterns. AI can identify subtle, leading indicators of potential disruptions that human analysts might easily miss or identify too late. For example, an AI could correlate a minor political protest in a key mineral-producing country (identified from news feeds) with unusual shipping delays (from logistics data) and a rising number of mentions of “shortage” on industry forums. It might then flag an impending supply chain risk for a specific raw material weeks, or even months, before it severely impacts production schedules and financial results.
This type of AI financial forecasting for risk management moves beyond relying purely on historical financial data. It actively incorporates these rich, external, often qualitative data sources, and helps to quantify their potential impact. This allows companies to build much more robust and dynamic contingency plans. They can proactively identify alternative suppliers. They can geographically diversify their operations. They can even adjust inventory levels based on forward-looking, AI-driven risk scores. For example, major logistics companies like DHL are leveraging AI and big data. They aim to anticipate disruptions and improve supply chain visibility for their clients. While DHL provides logistics services, the invaluable insights generated can be directly fed into their clients’ FP&A teams. These teams can then model the financial impact of such potential disruptions. The ability to integrate these advanced risk signals into financial models means FP&A teams can more accurately forecast the costs of mitigation strategies. They can assess the financial viability of different resilience measures. And they can better allocate resources using powerful AI in FP&A capabilities. This ultimately builds a more resilient and adaptable organization, ready to face future uncertainties.
Trend 3: The Rise of “Explainable AI” (XAI) and Ethical Considerations in Financial AI
Artificial intelligence can perform incredible analytical feats. It can identify complex patterns hidden deep within data that humans would likely overlook. It can generate highly accurate financial predictions. But there is often a nagging question from users: how exactly does the AI arrive at these answers? Many advanced AI models, particularly deep learning systems, can operate like “black boxes.” Data goes in, and a decision or prediction comes out. However, the internal reasoning process often remains opaque. This lack of transparency is a significant hurdle. It affects the widespread adoption and, crucially, the trust in AI in FP&A. Finance professionals are ultimately accountable for the numbers they present. They need to understand them. They also need to be able to explain them clearly to all stakeholders, from a departmental manager asking about a budget variance to the board of directors discussing strategic investments.
This is precisely why “Explainable AI,” or XAI, is rapidly becoming a critical emerging trend in the financial technology space, and indeed across all AI applications. XAI encompasses a set of techniques and methodological approaches. These are aimed at making AI models transparent and interpretable by human users. XAI allows users to peek inside the so-called “black box.” It helps them understand how the AI reached its specific conclusions or generated its forecasts.
For instance, if an AI financial forecasting tool flags a particular product line for potential divestment due to projected declining profitability, XAI could highlight the key contributing factors. Perhaps it’s a combination of rising material costs identified in supplier data. Or maybe it’s negative customer sentiment picked up from online product reviews. It could also be emerging competitor activity that the AI has detected.
This transparency is vital for several important reasons. Firstly, it builds trust among users. FP&A teams are far more likely to rely on AI-driven insights if they can understand the underlying logic. Secondly, it allows for proper validation and debugging of the AI models. If an AI produces an unexpected or counterintuitive result, XAI can help pinpoint whether it is a genuine, valuable insight or an error stemming from flawed input data or inherent model bias. Thirdly, ethical considerations are paramount in this field. AI tools used in finance can influence decisions that directly impact jobs, shape investment strategies, and determine strategic resource allocation. It is crucial to ensure these powerful systems are fair, unbiased, and auditable.
Financial regulators globally are also increasing their scrutiny of algorithmic decision-making. Companies like Fiddler AI, and others in the growing AI governance space, are developing platforms focused on model monitoring, explainability, and fairness detection. These tools help businesses understand, validate, and manage their AI systems responsibly. As AI in FP&A tools become more sophisticated and integral to day-to-day financial operations, the demand for robust XAI features and a strong ethical framework will only continue to grow. This will ensure AI serves as a trusted, transparent, and accountable partner in finance.
Embracing the Next Wave of Financial Intelligence
The world of corporate finance is clearly on the move, and AI is at the helm. AI in FP&A is no longer just a futuristic buzzword. It is a powerful, tangible force for change, already delivering value. We’ve explored some exciting new trends that go beyond simple automation. We saw how AI can offer hyper-personalized financial advice to managers. We looked at its growing power in predictive risk modeling through diverse data sources. And we discussed the crucial, rising need for explainable and ethical AI.
These trends clearly show that AI financial forecasting and AI-driven analysis are becoming significantly smarter, more insightful, and more accessible. For businesses ready to embrace these advancements, the future of their finance function looks very bright. Adopting these advanced AI applications is about more than just improving operational efficiency. It’s about building a finance team that truly understands the present business dynamics. It’s about creating a team that can better predict future challenges and opportunities. Ultimately, it’s about a team that drives smarter, more agile decisions across the entire organization. The journey with AI in finance is still in its early stages, but the potential is undeniably transformative.