In today’s fast-changing financial world, information is power. But how do you sift through mountains of financial disclosures and market news? This is where Natural Language Processing (NLP) comes in. NLP helps computers understand human language. When applied to finance, it can dig out valuable insights from text that humans might miss.
Traditional financial analysis often relies on numbers and structured data. However, a vast amount of crucial information is locked away in words. Think of regulatory filings, earnings call transcripts, news articles, and even social media posts. NLP and Artificial Intelligence (AI), particularly in the realm of AI market sentiment finance, are fundamentally changing this landscape. These technologies make it possible to analyze massive volumes of this unstructured text data at scale. This process uncovers subtle intelligence and actionable insights. This article looks into some less-discussed, emerging trends in this exciting field.
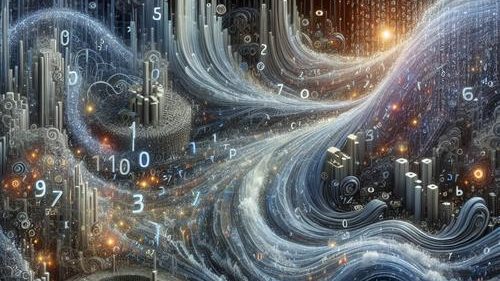
Uncovering Deeper Financial Trends with NLP
Many are familiar with NLP’s ability to track basic sentiment in stock news. But the real, evolving power of NLP lies in identifying deeper, less obvious trends and risks. Let’s explore some cutting-edge areas where sophisticated NLP financial analysis is making significant progress, moving far beyond simple positive or negative classifications.
Trend 1: Granular Analysis of Supply Chain Risks from Disclosures
Company financial reports, like annual 10-K filings, often contain sections discussing potential supply chain risks. Traditional analysis might involve searching for keywords such as “disruption” or “shortage.” However, advanced NLP offers a much more nuanced approach. It can understand the context surrounding these risk mentions. NLP models can identify specific suppliers, raw materials, or geographic regions that are repeatedly flagged or discussed with increasing concern.
Furthermore, NLP can detect subtle shifts in the language used by companies over successive reporting periods. For instance, a company’s language might evolve from mentioning “potential logistical challenges” in one quarter to “anticipated component scarcities” in the next. Such nuanced changes, often missed by human analysts reviewing documents in isolation, can signal escalating problems. This capability allows for early identification of vulnerabilities. Tracking how frequently a company discusses issues with critical partners or specific choke points in their supply network offers predictive power.
A practical example involves using NLP to scan global news, trade publications, and regulatory filings. This data is cross-referenced with a company’s disclosures. If a company heavily relies on a particular material sourced from a politically unstable region, NLP can flag this. It correlates news of emerging instability in that region with mentions of that material or supplier in the company’s filings. This action happens even if the company itself doesn’t explicitly label it a high-priority risk. This deep-dive form of NLP financial analysis is extremely valuable. Technology leaders like IBM provide underlying AI and NLP platforms that enable such sophisticated text analytics for risk management.
Trend 2: Gauging Employee Sentiment and Culture from Publicly Available Data
Employee morale and company culture can be surprisingly potent leading indicators. They often reflect a company’s internal health and can impact future operational performance. An often-overlooked source of insight lies in publicly available data. This includes employee reviews on platforms like Glassdoor, anonymous posts on professional forums such as Fishbowl, or even discussions on LinkedIn.
NLP algorithms can process vast quantities of this employee-generated text. They can gauge overall sentiment towards management, strategy, and work environment. These tools can identify recurring themes in complaints or praises. They can also spot trends in discussions about employee turnover, burnout, diversity and inclusion efforts, or positive mentions of innovation culture. A sudden increase in negative language related to company leadership or a pessimistic future outlook, identified by NLP and sentiment analysis tools, is a critical signal. This type of AI market sentiment finance application is growing.
This type of analysis acts as an early warning system. It can flag potential operational frictions or talent retention issues. These problems might surface long before they manifest in quarterly financial statements. This represents a less conventional but increasingly powerful application of NLP financial analysis. For instance, some specialized investment firms are known to use custom NLP models. These models analyze aggregated employee review data for patterns. They look for early signs of internal discord or declining morale at publicly traded companies. A sharp downturn in employee satisfaction scores, coupled with increased commentary about poor strategic decisions, could be a significant red flag for investors.
Trend 3: Identifying Novel Thematic Investments from Diverse Textual Data Lakes
Investors are perpetually searching for “the next big thing.” They aim to identify broad, thematic investment opportunities that promise long-term growth. Examples include the accelerating transition to renewable energy, shifts in global manufacturing driven by geopolitical factors, or evolving consumer behaviors fueled by generative AI. Pinpointing these nascent themes and their potential financial impact solely from structured financial data is exceptionally difficult. This is where another emerging trend in NLP financial analysis shines.
NLP can systematically scan and interpret massive “textual data lakes.” These repositories consist of diverse unstructured and semi-structured content. This includes global news feeds, academic research papers, patent filings, transcripts from industry conferences, corporate earnings calls, and even relevant social media discussions. Sophisticated NLP algorithms identify frequently recurring concepts and, more importantly, the relationships and connections between them across these varied sources. This helps in spotting emerging narratives, new technologies, or shifting policy landscapes that could drive future market movements. It forms a cornerstone of advanced AI market sentiment finance.
For example, an NLP system might detect an increasing co-occurrence of terms. These could relate to new solid-state battery breakthroughs, government initiatives for EV charging infrastructure, and rising consumer search interest for electric vehicles. By piecing together these scattered signals from thousands of documents, NLP can construct a data-backed thesis for an emerging investment theme. This offers a clearer and more timely picture than manual research alone. Major financial data providers like Refinitiv (an LSEG business) and Bloomberg are continuously enhancing their offerings with NLP-powered tools. These tools assist financial professionals in identifying, monitoring, and analyzing such thematic investment opportunities.
Conclusion: The Intelligent Future of Financial Analysis
Natural Language Processing is undeniably revolutionizing how we approach and interpret financial information. It empowers analysts, investors, and risk managers to unlock profound insights. These insights are often concealed within the colossal and ever-growing ocean of unstructured text. By moving significantly beyond rudimentary keyword searches and basic positive/negative sentiment scores, NLP is adept at revealing more subtle, predictive, and ultimately actionable signals from text.
We’ve explored how it can meticulously identify nuanced supply chain risks before they critically impact operations. We’ve seen its capacity to gauge internal company health and culture by “listening” to employee voices across public platforms. Furthermore, NLP is proving invaluable in spotting nascent thematic investments by connecting disparate pieces of information into a coherent narrative. These advanced applications of NLP financial analysis provide a substantial competitive advantage in today’s dynamic and data-intensive markets. As NLP technology and AI algorithms continue to evolve in sophistication and accessibility, their role in extracting strategically vital intelligence from financial disclosures and market news will only become more central. This truly marks the exciting and intelligent future for both comprehensive AI market sentiment finance and broader financial analytics.